Book
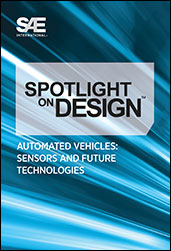
Automated Vehicles: Sensors and Future Technologies (DVD)
2015-04-15
"Spotlight on Design" features video interviews and case study segments, focusing on the latest technology breakthroughs. Viewers are virtually taken to labs and research centers to learn how design engineers are enhancing product performance/reliability, reducing cost, improving quality, safety or environmental impact, and achieving regulatory compliance. In the episode "Automated Vehicles: Sensors and Future Technologies" (24:31), highly automated driving is looked at in detail as the culmination of years of research in automotive technology, sensors, infrastructure, software, and systems integration. Real-life case studies show how organizations are actually developing solutions to the challenge of making cars safer with less driver intervention. IAV Automotive Engineering demonstrates how a highly automated vehicle capable of lane changing was created.